AI-Assisted “Vibe” Coding - For Work / Play
I began exploring vibecoding for both my personal projects and Inferless, and I experienced firsthand how it enhanced both. In technical terms "Vibe" coding is an AI-assisted software development approach where you describe the desired functionality in natural language, and AI tools generate the corresponding code. After several months of experimentation, I believe this represents the most significant paradigm shift in the software development lifecycle.
So what changes with vibe coding ?
For Work Projects :
- Enhanced Project Understanding: AI-assisted chat tools help break down even complex codebases, making it easier to grasp the underlying logic—even when you're not fully familiar with every language detail.
- More Confident Code Changes: By utilizing AI to navigate through project dependencies and code structures, you can more readily identify which areas may be affected by changes, leading to more confident and precise modifications.
- Accelerated Feature Development: AI s treamlines the integration of new features by quickly parsing documentation for SDKs or APIs, enabling faster and more efficient support for additional functionalities.
Fiddling with Hobby Projects :
- Rapid Prototyping: I find it extremely valuable in hackathons, where an initial prompt can be quickly transformed into a basic MVP. The challenge, however, lies in refining the prompt enough to produce a truly polished MVP.
- Bridging Language Barriers: A significant advantage of vibecoding is its ability to effortlessly translate an algorithm or feature into the programming language best suited for the project.
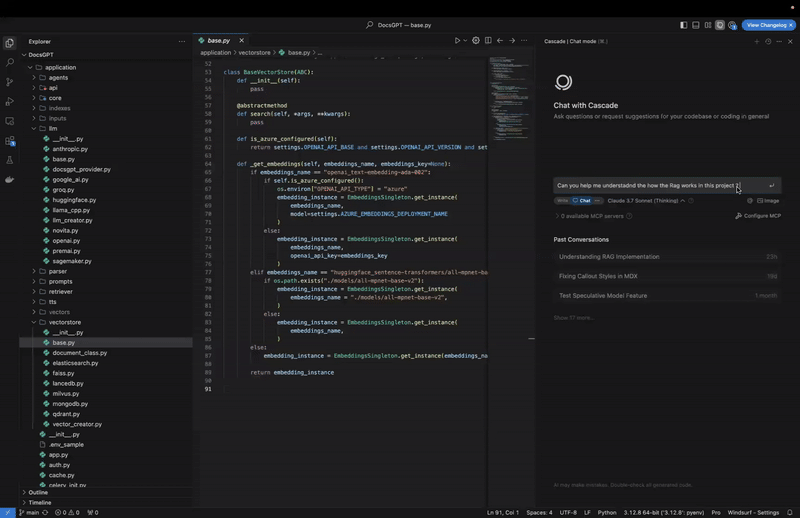
While vibe coding has several advantages it does come with caution and if not handled well can lead to a code base that has pretty bad code quality and very high security vulnerability. There is a need for systems that need to keep that Vibe in Check.
Keeping the "Vibe in Check"
With vibe-coding there is influx of code that can come into the system which might make it super hard to fix and debug if it goes wrong. Here are a best practice I have been using when you are rapidly iterating
- Building efficient rules files: An efficient rules file encapsulates the project's overall purpose, key features, and domain-specific constraints to provide the AI with essential context, while detailing coding standards—such as naming conventions and formatting rules—to ensure uniform, maintainable code. It also outlines the desired architecture and file organization for coherent multi-file navigation, specifies technical preferences like language features, frameworks, API versioning, and dependency management, and establishes clear boundaries for sensitive files to safeguard against unintended modifications.
- Take Frequent Snapshots and Review: Regularly taking snapshots—essentially, saving versions of your code—is a powerful safety net with Videcoding. Frequent reviews of these snapshots help catch any unintended side effects early on, ensuring that the integration of new code doesn't introduce hidden bugs.
- Make small and iterative changes : By iterating in manageable steps, you reduce the risk of introducing multiple errors simultaneously. This approach not only makes troubleshooting easier but also allows you to validate each change in isolation.
- Manual Code Review : <anual code review remains crucial. A human perspective is necessary to catch nuances or context-specific issues that an AI might overlook. Like checking for hardcoded strings, too complex code and the library version check ( this is often outdated ).
One of the most important things for the vide coding to pass the context of the project and directory structure so that it now where to find the code and how to create the new code into.
Tools and Models for Coding
I have been evaluating both Windsurf and Cursor and using gpt-o3-mini calude 3.5 and Claude 3.7 for various scopes for the projects. Both of them have been super good, but I have found various aspects of the coding where some of their combinations are better
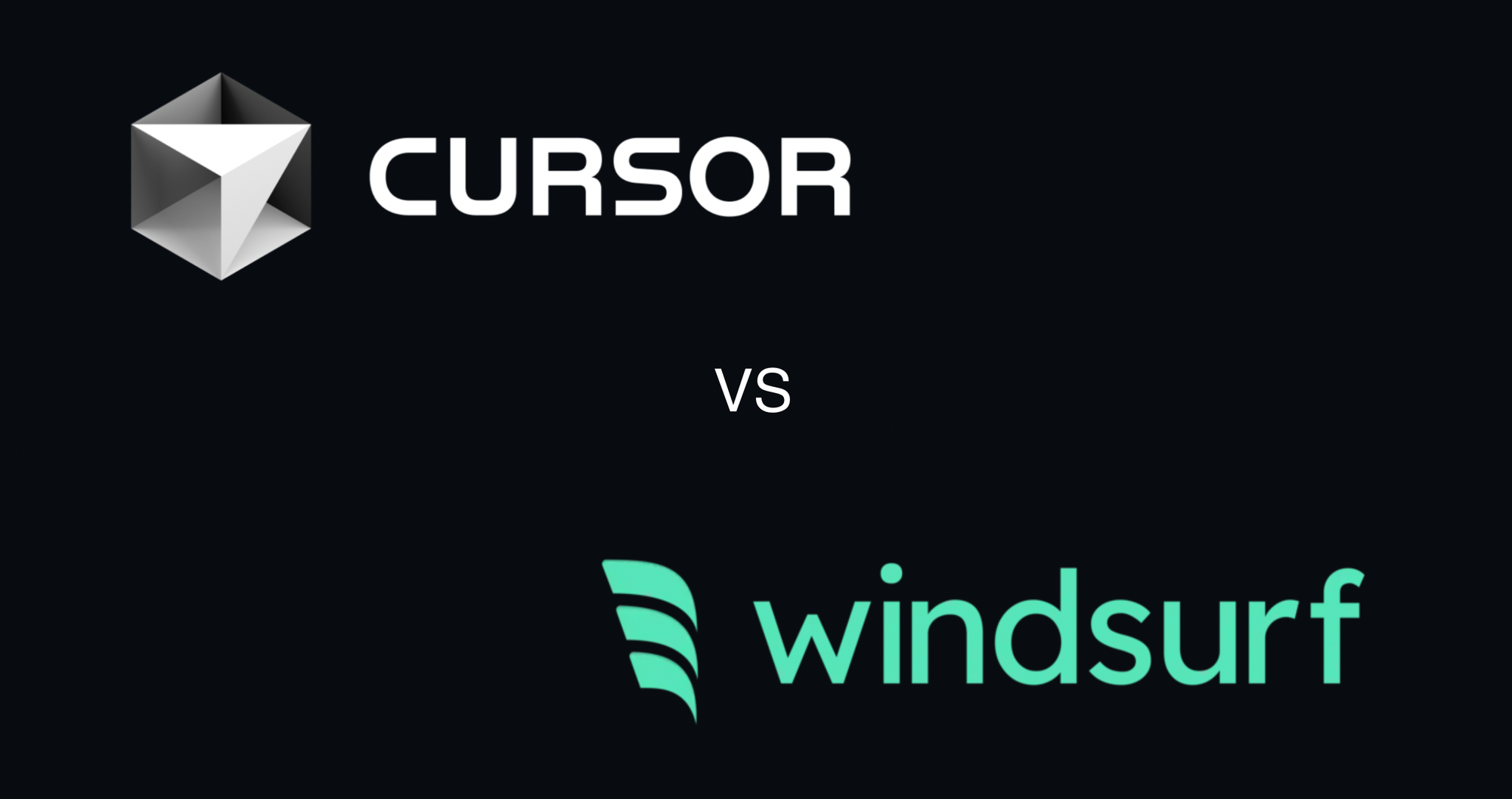
- Scaffolding New Projects:
For hackathons or starting projects from scratch, combining Claude 3.7 with Cursor proves to be the most effective. This pairing excels in rapid code generation, delivering a high-quality MVP and offering an outstanding experience in creating fresh code. - Making Edits for Large Projects:
When working on large projects, Windsurf paired with Claude 3.5 outperforms other combinations due to its advanced indexing and pre-computation capabilities that quickly identify relevant files. In contrast, using Cursor for similar tasks often requires more precise and detailed prompts. - Complex Thinking and Multi-Step Changes:
For tackling complex, multi-step changes, the optimal setup is Cursor combined with Claude 3.7 in thinking mode (or with a gpt-o3-mini configuration). This configuration is particularly adept at developing comprehensive features that demand intricate, well-planned modifications.
Again there are experiences after a lot of hit and trial and trying to solve the task type with multiple editors.
The Future
Here are some new challenges and opportunities that are still left that I think the future for "Videcoding" to improve
- Support for Regression-based learning: One of the major improvements that see opportunity is the ability to learn from past mistakes. I see a lot of times when the model makes an incorrect change, sometimes even after the prompt it continues to go back to the incorrect solution. I see if there is context on what was wrong and keep that in context for the next change. Also, we can use RL to generate a better answer.
- Support for Better Visual Orchestration for Components: For most of the visual components I still see images as the only way to interface for UI development. I see an opportunity where the visual editor can effectively adjust
- Model Context Protocol for data for ingestion and AI Tools : Extending AI Behavior with External Toolsis an open standard that lets AI models connect to external tools and data sources in a consistent way, MCP can send requests to MCP servers (each server providing access to a particular tool or service) and use their responses in its output
- Web APIs and Data – e.g. a Google Maps MCP server to get map or location data. You might ask “Find the distance between two addresses,” and Cascade will call the Google Maps tool. It cloud also connect with posthog to get the logs / exception for the
- Developer Services – e.g. a GitHub MCP server to create issues or commit code via GitHub’s API, or a Supabase/Postgres server to run SQL queries on a database.
- Internal/External Tools – You can even connect internal systems. shell/terminal MCP server could let the AI run safe terminal commands. Zapier MCP tool would let Cascade do things like create tickets in Jira, send Slack messages, or update a Google Sheet
This greatly expands what “AI-assisted development” means: the AI isn’t just writing code you ask for, but can also execute tasks on your behalf (setting up environment, updating external resources, etc.), acting like a junior developer with superpowers to operate various services.
I am excited to see what comes next to enhance the developer productivity